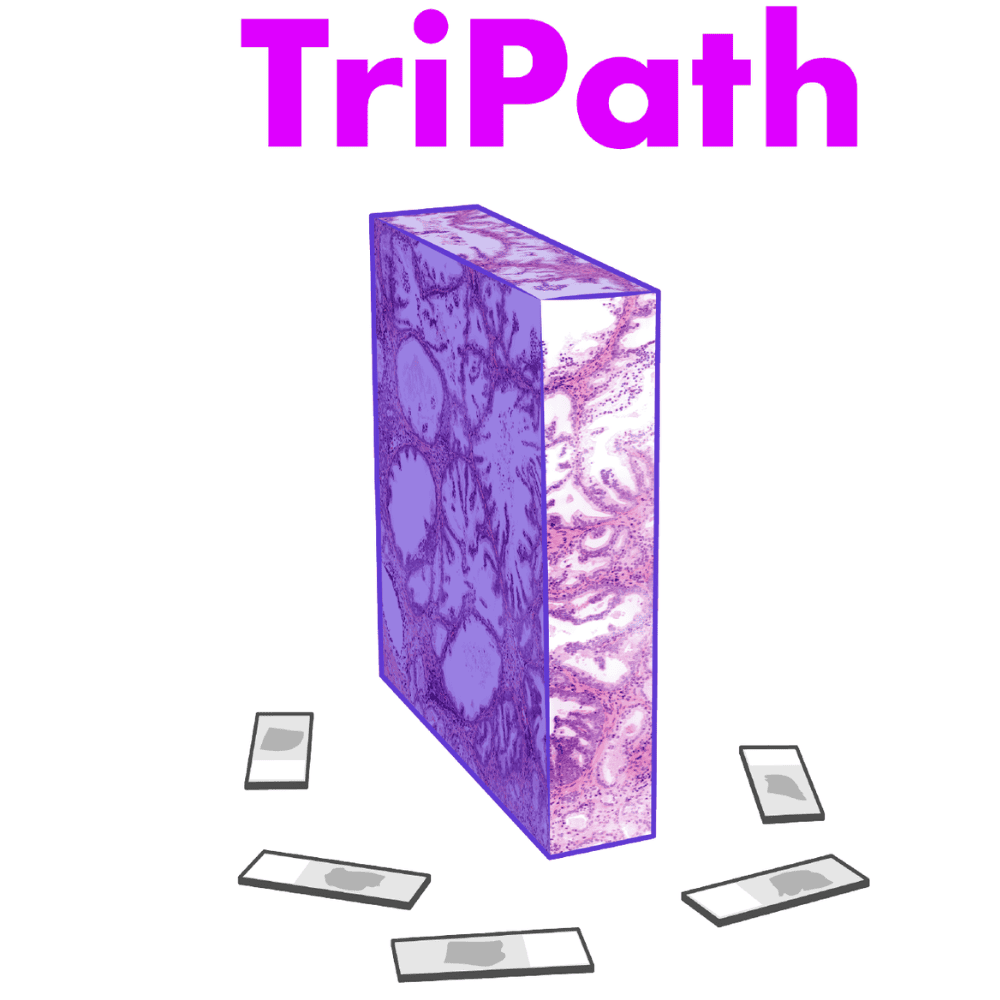
The analysis of human tissue samples—taking thin slices, staining them, mounting them on slides, and viewing them through a microscope—hasn’t fundamentally changed for more than a century. While this technique can help diagnose and predict disease progression, it is inherently limited by its two-dimensional nature. Humans, after all, aren’t flat, and 2D image slices can only tell us so much about our 3D bodies (and tissues contained therein).
3D pathology techniques have recently emerged, but their use in a clinical setting poses several challenges. Notably, the information captured from high-resolution 3D samples is enormously more complex than what is gathered from traditional 2D image slices. Analyzing 3D tissue datasets manually is nearly impossible and important details that could help improve prognosis are likely missed.
A team of NIBIB-funded researchers recently developed TriPath, an AI platform that can analyze 3D pathology images to predict disease outcomes. Their method, reported in the journal Cell, had improved performance in predicting prostate cancer outcomes when compared with traditional pathology approaches, such as analysis by expert pathologists using 2D images.